Synthesizing New Proteins: AI Model Creates Custom Proteins Beyond Nature
The field of synthesizing new proteins, the building blocks of biological life, is experiencing a revolution with the development of a groundbreaking AI model. This new model promises to provide instructions for creating proteins that go far beyond what is naturally found in living organisms.
Recently, scientists in the US utilized EvolutionaryScale Model 3 (ESM3) to design a novel protein named esmGFP (green fluorescent protein). This protein only shares 58 percent of its composition with its closest natural relative, tagRFP.
According to the research team, this is equivalent to 500 million years of evolution processed by AI. This breakthrough opens up possibilities for designing custom-made proteins tailored for specific applications and unlocking new functionalities from existing proteins.
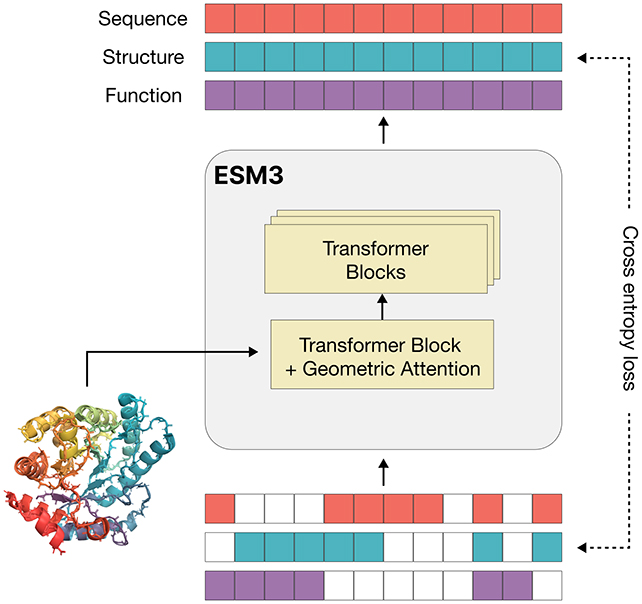
The researchers, led by Thomas Hayes, founder of EvolutionaryScale in New York, explained in their paper that more than three billion years of evolution have shaped the biological image encoded in natural proteins. They demonstrated that language models trained on evolutionary data can generate functional proteins that are vastly different from known proteins.
I’m so excited to share what we’ve been working on @EvoscaleAI. ESM3 is a multimodal generative masked language model for programming biology. Here’s a short thread on the architecture behind ESM3. 🧵https://t.co/jldHYRAPNy
— Thomas Hayes (@THayes427) June 25, 2024
ESM3 was trained on an extensive dataset comprising 3.15 billion protein sequences, 236 million protein structures, and 539 million protein annotations. By analyzing patterns in this vast data, the AI model can discern effective protein building strategies and functions.
What sets esmGFP apart is its functionality – it fluoresces like tagRFP. Fluorescent proteins play a crucial role in various applications, including as markers in medicine and biotechnology, and even giving certain ocean organisms their luminescence.
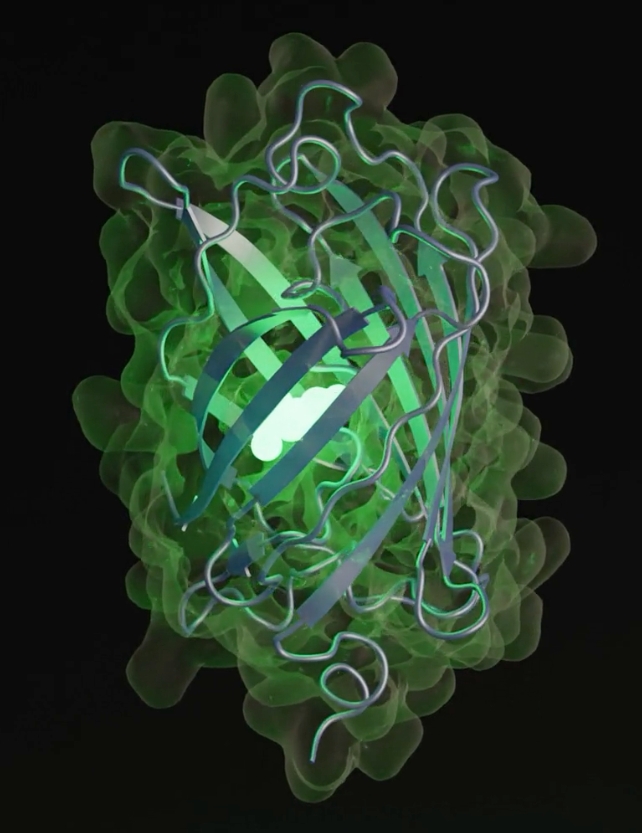
The AI model streamlines the process of protein synthesis by minimizing trial and error while enabling exploration of uncharted territories in protein design.
The researchers emphasized that proteins exist within an organized space where each protein is connected to every other protein through potential evolutionary pathways. ESM3’s design is based on recognizing proteins in this space.
While proteins designed by ESM3 require validation, synthesis, and testing, the team is optimistic about further advancements in this field. In the near future, we could witness the production of proteins for a wide range of applications, from medicines to biomaterials, with the assistance of sophisticated AI algorithms.
The researchers explained that protein language models construct a model of the myriad potential evolutionary paths that could have been followed, rather than working within the physical constraints of evolution.
This groundbreaking research has been published in Science.